Bastien Doignies: SIGGRAPH Asia 2023 Research
Can algorithms be art? Bastien Doignies's work suggests they can be, blurring the lines between computational processes and creative expression. His explorations in computer graphics, particularly using diffusion models to generate point clouds, offer a fascinating glimpse into the future of digital art and its potential to revolutionize fields beyond aesthetics.
Doignies's research, presented at Siggraph Asia 2023 in Sydney, Australia, and detailed in the forthcoming ACM Transactions on Graphics (2024), delves into a novel method for creating point clouds sets of data points in space which are fundamental building blocks for 3D modeling and computer graphics. Traditionally, creating these point clouds has been a laborious process, often relying on manual input or complex algorithms. Doignies's innovative approach leverages the power of diffusion models, a type of machine learning model that has recently gained significant traction in image generation. These models, known for their ability to create stunningly realistic and diverse images, are now being explored for their potential in other domains, thanks to researchers like Doignies.
Bio Data & Personal Information | Career & Professional Information |
---|---|
Name: Bastien Doignies Born: June 6, 1992, Maubeuge, France Nationality: French | Researcher at Universit Claude Bernard Lyon 1, CNRS, INSA Lyon, LIRIS Focus: Computer Graphics, Diffusion Models, Point Cloud Generation Collaborators: David Coeurjolly, Nils Krah, Jean Michel Ltang, Victor Ostromoukhov, David Sarrut, and others. |
Learn more about Bastien Doignies's publications.
Differentiable Owen scrambling, a key aspect of Doignies's work, allows for greater control and manipulation of these diffusion models. This differentiability is crucial, enabling the algorithm to learn and adapt, ultimately leading to more nuanced and intricate point cloud generation. By applying these techniques, Doignies opens up exciting possibilities for creating complex 3D models with unprecedented ease and precision.
Beyond the realm of pure aesthetics, the implications of this research extend to various practical applications. In medical simulations, for instance, advanced sampling techniques, including those using machine learning approaches like Doigniess, can significantly enhance the accuracy and efficiency of Monte Carlo simulations. These simulations are vital tools for modeling complex systems in fields ranging from nuclear physics and finance to weather forecasting and, notably, medical applications. The International Conference on Monte Carlo Techniques for Medical Applications (MCMA) 2022, held in Antwerp, Belgium, highlighted the growing importance of these methods in healthcare.
Traditional Monte Carlo samplers can be computationally expensive, often relying on slow optimization processes and a wide range of numerical methods. Moreover, they are not always differentiable, limiting their adaptability and integration with modern machine learning techniques. Doigniess work addresses these limitations, providing a pathway towards more efficient and flexible samplers. His research contributes to the ongoing development of more robust and powerful tools for medical simulations, potentially leading to more accurate diagnoses and personalized treatment plans.
Furthermore, the collaborative nature of Doignies's research, as evidenced by his co-authors and participation in groups like the "Groupe de Lecture Origami," underscores the importance of open communication and knowledge sharing in advancing scientific discovery. These regular meetings provide a platform for researchers to discuss and critique each other's work, fostering a dynamic environment for innovation.
Doignies's work also reflects a broader trend in the computer graphics community, where researchers are increasingly leveraging the power of AI and machine learning to push the boundaries of what's possible. The success of recent diffusion models in image generation has sparked a wave of interest in their application to other areas, including 3D modeling and animation. Doignies's innovative approach to point cloud generation positions him at the forefront of this exciting new frontier. He continues to explore the creative potential of algorithms, transforming complex mathematical processes into tools for artistic expression and scientific advancement.
His work with Eulerian methods for inverse problems using optimal transport, supported by the ANR ROOT project, further demonstrates his commitment to tackling complex challenges in computer graphics. This research has the potential to improve the accuracy and efficiency of various applications, including medical imaging and 3D reconstruction.
While his research is complex, Doigniess commitment to sharing his work and collaborating with others demonstrates his dedication to pushing the field forward. His presentations at conferences like Siggraph Asia, publications in respected journals, and engagement with the broader research community through platforms like GitHub (where he builds software under the username minipattoune) contribute to a growing body of knowledge that benefits both researchers and practitioners in the field.
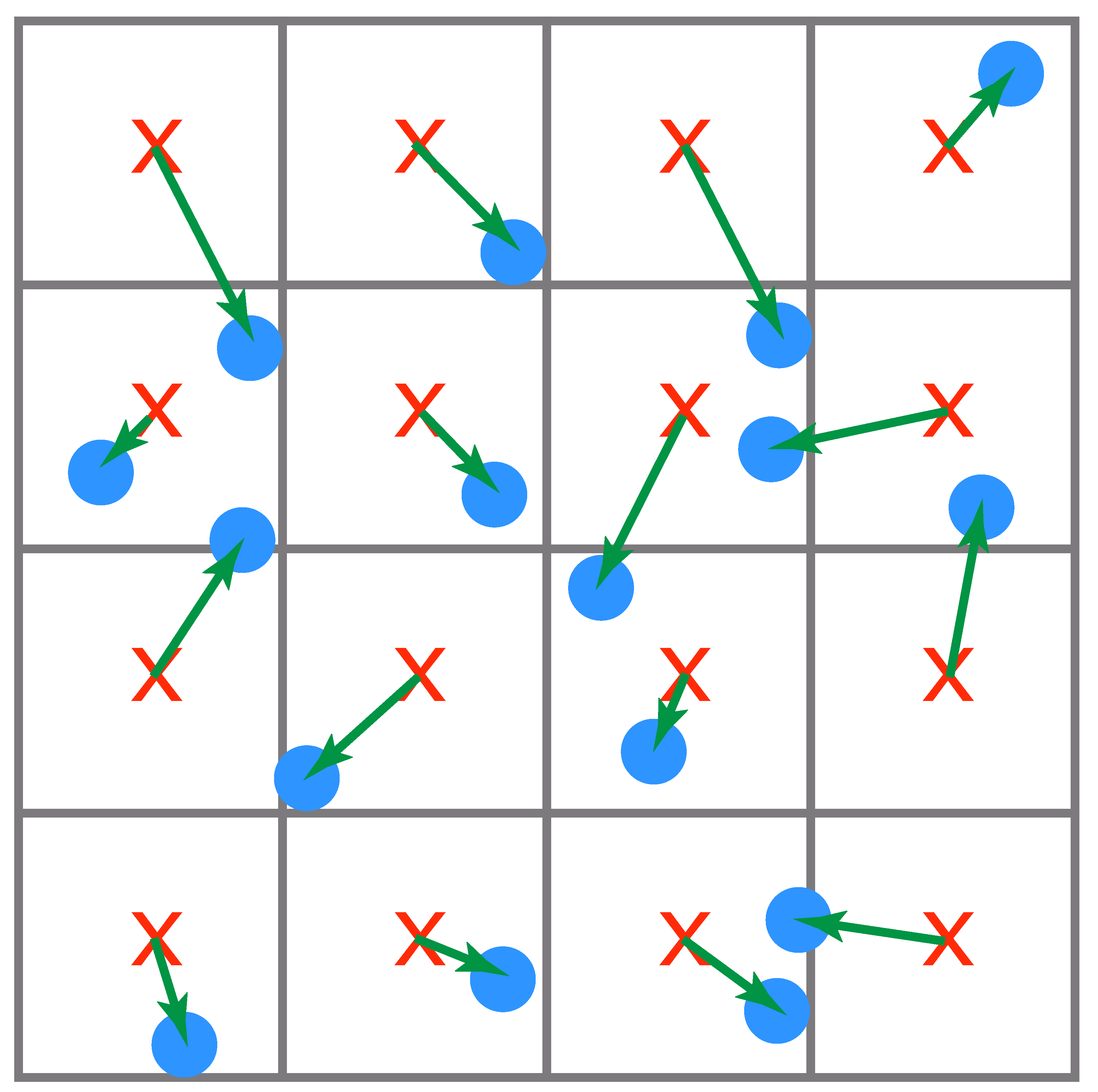
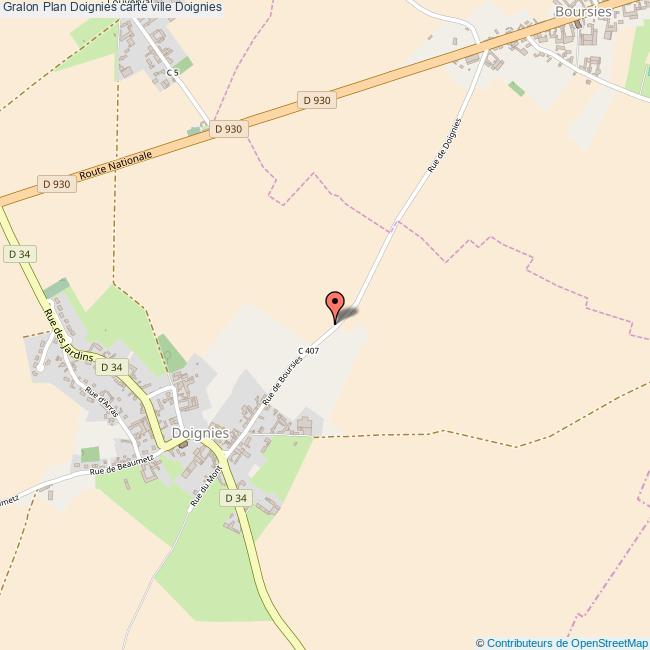
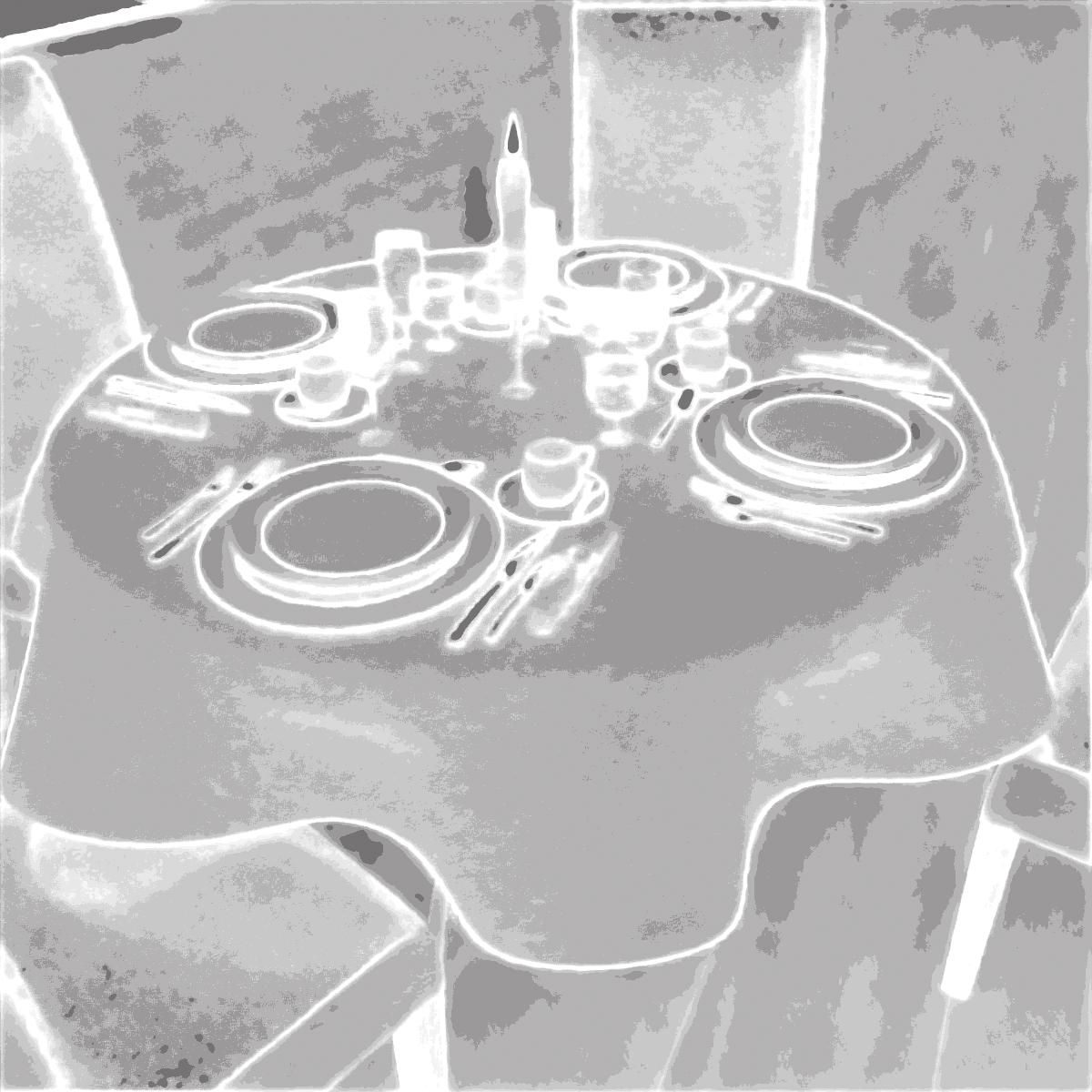